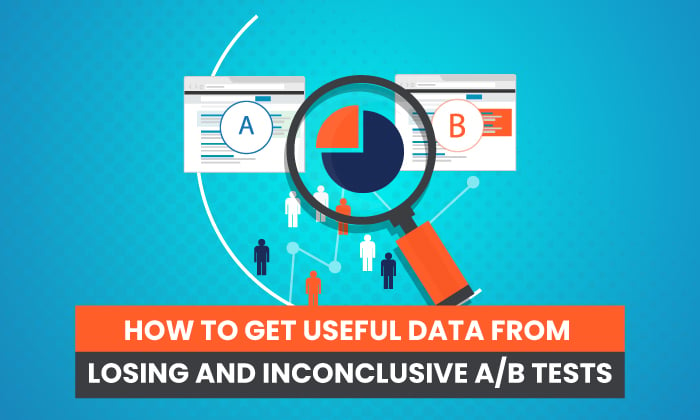
A/B testing is crucial to developing a robust digital marketing strategy. However, not all tests result in valuable data.
What do you do if a variation you thought would rock ends up flopping? Or what if your test results are inconclusive?
Don’t throw in the towel just yet!
There’s a ton you can do with inconclusive or losing A/B testing data. We’re going to cover how to put that information to good use—but first, let’s cover why A/B testing matters in digital marketing.
Why A/B Testing Is Crucial to Digital Marketing Success
A/B testing helps marketers understand the impact of optimization methods. For example, it can show how changing an ad headline impacts conversions or whether using questions in titles drives more traffic.
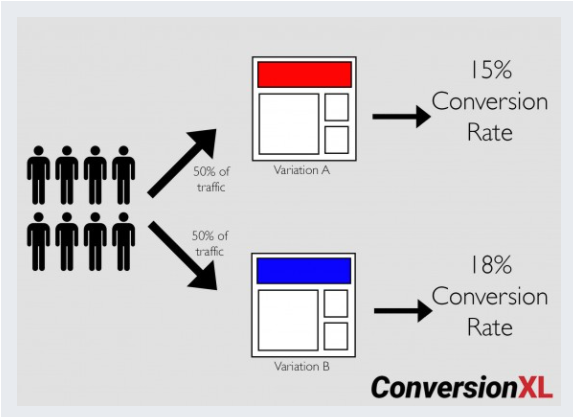
A/B testing provides hard data to back up your optimization techniques. This allows marketers to make better business decisions because they aren’t just guessing at what drives ROI. Instead, they’re making decisions based on how specific changes impact traffic, sales, and ROI.
How Do I Know If I Have a Losing or Inconclusive A/B Test?
After running an A/B test, you’ll see the results in your own data dashboard (such as Google Analytics) or in the testing tool you use.
Optimizely, a popular A/B testing platform, provides data in an experiment results page, which tracks each variation, number of visitors, how many people completed a specific action, revenue, and other metrics.
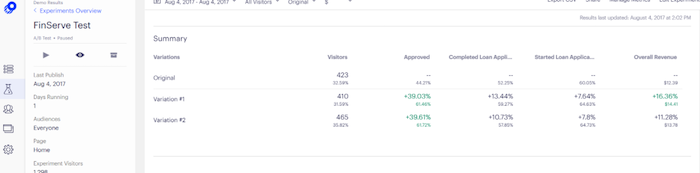
The example above shows variation number one had fewer visitors but drove 5 percent more revenue, making it a clear winner.
Other times, the numbers might be much closer. An inconclusive test might mean the numbers are less than a percent off, or neither variation got any traffic at all.
When your tests don’t have enough data or if the numbers are too close, they are considered inconclusive or statistically insignificant.
Then, use these tips to make the most of your data.
6 Ways to Leverage Data From Losing or Inconclusive A/B Testing
You’ve run your A/B tests and are excited to get the results. Then, something unexpected happens: The variation you expected to win performs worse! Or you find the variations don’t actually impact the metrics you are tracking at all.
Now what? Don’t assume your test failed. There are plenty of steps you can take to leverage that data.
Try Something Really Different
Inconclusive test results could mean your variations are too close. A/B testing can help you see if a small change (like using red versus green buttons) impacts conversions, but sometimes those tiny tweaks don’t have much impact at all.
Remember that you may need to run the test with several similar variations to see what caused the change.
Rather than getting discouraged, consider it an opportunity to try something totally different. For example, change the page layout, add a different image or take one away, or completely revamp your ad, asset, or CTA.
Analyze Different Traffic Segments
So, your A/B test came back with almost identical results. Does that mean nothing changed? Maybe not. Rather than looking at all the data, try segmenting the audience to see if different people responded differently.
For example, you might compare data for:
- new versus returning customers
- buyers versus prospects
- specific pages visited
- devices used
- demographic variations
- locations or languages
Overall, your test might be inconclusive. However, you might find specific segments of your audience respond better to certain formats, colors, or wording.
You can use that information to segment ads more appropriately or create more personalized ads or content.
Look Beyond Your Core Metrics
Conversions matter, but they aren’t everything. You might have hidden data in your losing test results.
For example, you might find conversions were low, but visitors clicked to view your blog or stayed on the page longer.
Sure, you may rather have sales. However, if visitors are going to read your blog it means you’ve connected with them somehow. How can you use that information to improve the buying process?
Say you run two variations of an ad. If one variation drives massive traffic, and 30 percent of visitors from that variation convert, this could mean more revenue. Obviously the winner, right?
Not necessarily. Take a glance at your “losing” ad to see if it drove less traffic but had higher conversions, for instance. If you’d only been looking at traffic and outright revenue, you might not have noticed the second ad works better statistically, if not in rough numbers.
Now, you can dig into the data to find out why it drove less traffic and use that to improve your next set of ads.
Remove Junk Data
Sometimes tests are inconclusive not because your variations were terrible or your testing was flawed, but because there’s a bunch of junk data skewing your results. Getting rid of junk data can help you see trends more clearly and drill down to find crucial trends.
Here are a few ways to clean up junk data so you can get a clearer understanding of your results:
- Get rid of bot traffic.
- If you have access to IP addresses, remove any from your company IP address.
- Remove competitor traffic, if possible.
Also, make sure to double-check tracking tools you use, such as URL parameters, work correctly. Failure to properly track testing can skew the results. Then, verify that sign-up forms, links, and anything else that could affect your data are in working order.
Look for Biases and Get Rid of Them
Biases are external factors impacting the results of your test.
For example, suppose you wanted to survey your audience, but the link only worked on a desktop computer. In that case, you’d have a sample bias, as only people with a desktop will respond. No mobile users allowed.
The same biases can impact A/B tests. While you can’t get rid of them entirely, you can analyze data to minimize their impact.
Start by looking for factors that could have impacted your test. For example:
- Did you run a promotion?
- Was it during a traditionally busy or slow season in your industry?
- Did a competitor’s launch impact your tests?
Then, look for ways to separate your results from those impacts. If you can’t figure out what went wrong, try rerunning the test.
Also, take a look at how your test was run. For example, did you randomize who saw which versions? Was one version mobile-optimized while the other wasn’t? While you can’t correct these issues with the current data set, you can improve your next A/B test.
Run Your A/B Tests Again
A/B testing is not a one-and-done test. The goal of A/B testing is to continuously improve your site’s performance, ads, or content. The only way to constantly improve is to continually test.
Once you’ve completed one test and determined a winner (or determined there was no winner!), it’s time to test again. Try to avoid testing multiple changes simultaneously (called multivariate testing), as this makes it hard to see which change impacted your results.
Instead, run changes one at a time. For example, you might run one A/B test to find the best headline, another to find the best image, and a third to find the best offer.
Losing and Inconclusive A/B Testing: Frequently Asked Questions
We’ve covered what to do when you have losing or inconclusive A/B testing results, but you might still have questions. Here are answers to the most commonly asked questions about A/B testing.
What is A/B testing?
A/B testing shows different visitors different versions of the same online asset, such as an ad, social media post, website banner, hero image, landing page, or CTA button. The goal is to better understand which version results in more conversions, ROI, sales, or other metrics important to your business.
What does an inconclusive A/B test mean?
It can mean several things. For example, it might mean you don’t have enough data, your test didn’t run long enough, your variations were too similar, or you need to look at the data more closely.
What is the purpose of an A/B test?
The purpose of an A/B test is to see which version of an ad, website, content, landing page, or other digital asset performs better than another. Digital marketers use A/B testing to optimize their digital marketing strategies.
Are A/B tests better than multivariate tests?
One is not better than the other because A/B and multivariate tests serve different purposes. A/B tests are used to test small changes, such as the color of a CTA button or a subheading. Meanwhile, multivariate tests compare multiple variables and provide information about how the changes interact with each other.
For example, you might use multivariate testing to see if changing the entire layout of a landing page impacts conversions and which changes impact conversion the most.
What are the best A/B testing tools?
There are a wide range of testing tools based on your needs and the platform you use. Google offers a free A/B testing tool called Google Optimize. Paid A/B tools include Optimizely, VWO, Adobe Target, and AB Tasty.
You may also be able to run A/B tests using WordPress plugins, your website platform, or marketing tools like HubSpot.
Conclusion: Make the Most of Losing or Inconclusive A/B Testing
A/B testing is crucial to the success of your online marketing strategy. Whether you focus on SEO, social media, content marketing, or paid ads, you need A/B testing to understand which strategies drive results.
Every A/B test is valuable—whether your new variation wins, loses, or is inconclusive, there is important data in every test result. The steps above will help you better understand your A/B testing results so you can make changes with confidence.
Have you used losing or inconclusive A/B testing before? What insights have you gathered?
from Neil Patel https://ift.tt/3v6aaTO